Citation:
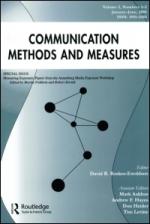
Abstract:
Given the scale of digital communication, researchers face a painful trade-off between powerful, scalable computational strategies, and the theoretical sensitivity offered by small-scale manual analyses. Especially in the study of natural discourse on digital media, the interactive, ever-evolving stream of conversations across multiple platforms regularly defies efforts to obtain well-defined samples of manageable size, while their linguistic variability imposes major limitations upon the accuracy of automated tools. In this paper, we draw upon recent advances in computational text analysis to develop a hybrid approach to the deductive analysis of large-scale digital discourse, which combines the algorithmic extraction of coherent, recurrent patterns with a manual coding of identified patterns. The approach scales up to treat millions of texts at minimal added human effort, while affording researchers close control over the process of theory-guided classification. We demonstrate the power of Hybrid Content Analysis by studying polarization in a quarter of a million contributions from cross-platform interactive social media discourse about a controversial incident.